About this Course
Advanced Deep Learning & AI Model Optimization
This course delves into the cutting-edge techniques for building, training, and optimizing sophisticated deep learning models. It's designed for individuals with a foundational understanding of machine learning and deep learning who are seeking to advance their skills and tackle real-world problems requiring high-performance AI solutions. The course emphasizes both theoretical understanding and practical application, providing you with the knowledge and abilities to design, implement, and deploy efficient and effective deep learning models across diverse domains.
Course Overview
This advanced course focuses on the critical aspects of deep learning beyond the introductory level. We explore advanced architectures, optimization strategies, and deployment techniques that are essential for building robust, scalable, and high-performing AI systems. You'll learn how to diagnose and address common challenges in deep learning, such as overfitting, vanishing gradients, and computational bottlenecks. The course also covers strategies for model compression, quantization, and deployment on resource-constrained devices.
Learning Objectives
- Understand and implement advanced deep learning architectures, including transformers, GANs, and graph neural networks.
- Master advanced optimization algorithms and techniques for faster convergence and improved model performance.
- Develop strategies for regularizing deep learning models and preventing overfitting.
- Learn techniques for model compression, quantization, and deployment on edge devices.
- Gain proficiency in diagnosing and addressing common challenges in deep learning, such as vanishing gradients and exploding gradients.
- Apply deep learning techniques to solve complex real-world problems across various domains.
- Evaluate the performance of deep learning models using appropriate metrics and methodologies.
- Stay up-to-date with the latest advancements and research trends in deep learning.
- Become proficient in using industry-standard deep learning frameworks such as TensorFlow and PyTorch for advanced model building and optimization.
Key Topics Covered
Advanced Deep Learning Architectures
- Transformers and Attention Mechanisms: Explore the architecture of transformers, including self-attention and multi-head attention, and their applications in natural language processing and computer vision.
- Generative Adversarial Networks (GANs): Dive into the theory and implementation of GANs for generating realistic data, image synthesis, and other creative applications.
- Graph Neural Networks (GNNs): Learn about GNNs for processing graph-structured data and their applications in social network analysis, drug discovery, and recommendation systems.
- Recurrent Neural Networks (RNNs) and LSTMs: Comprehensive study of RNNs and their variants like LSTMs and GRUs to understand sequential data handling, language modeling, and time series prediction.
- Autoencoders and Variational Autoencoders (VAEs): Examination of autoencoders and VAEs for dimensionality reduction, anomaly detection, and generative modeling.
Optimization Techniques
- Advanced Optimization Algorithms: Explore optimization algorithms beyond stochastic gradient descent (SGD), such as Adam, RMSprop, and Adagrad, and their adaptive learning rate strategies.
- Learning Rate Scheduling: Learn how to schedule the learning rate during training to improve convergence and model performance.
- Batch Normalization and Layer Normalization: Understand the benefits of batch normalization and layer normalization for stabilizing training and accelerating convergence.
- Gradient Clipping: Implement gradient clipping to prevent exploding gradients and improve training stability.
Regularization Strategies
- Dropout and DropConnect: Apply dropout and dropconnect techniques to prevent overfitting and improve model generalization.
- Weight Decay and L1/L2 Regularization: Use weight decay and L1/L2 regularization to penalize complex models and promote simpler solutions.
- Early Stopping: Implement early stopping based on validation set performance to prevent overfitting.
- Data Augmentation Techniques: Exploration of diverse data augmentation methods to artificially increase the size of the training dataset and improve model robustness.
Model Compression and Quantization
- Pruning: Learn how to prune redundant connections in deep learning models to reduce their size and complexity.
- Quantization: Apply quantization techniques to reduce the precision of model weights and activations, enabling deployment on resource-constrained devices.
- Knowledge Distillation: Transfer knowledge from a large, complex model to a smaller, more efficient model.
- Efficient Architectures (MobileNets, EfficientNets): In-depth analysis of efficient architectures designed for mobile and embedded devices, optimizing for both accuracy and computational efficiency.
Deployment and Scalability
- Model Serving: Learn how to deploy deep learning models using popular serving frameworks such as TensorFlow Serving and TorchServe.
- Edge Deployment: Deploy deep learning models on edge devices such as smartphones and embedded systems.
- Scalable Training: Explore techniques for distributed training and parallel processing to accelerate model training on large datasets.
- Cloud Deployment Strategies (AWS, GCP, Azure): Examination of different cloud-based solutions and strategies for deploying deep learning models on platforms like AWS, Google Cloud Platform, and Azure.
Advanced Topics
- Explainable AI (XAI): Understand techniques for interpreting and explaining the decisions made by deep learning models.
- Adversarial Attacks and Defense: Learn about adversarial attacks that can fool deep learning models and techniques for defending against them.
- Federated Learning: Explore federated learning for training deep learning models on decentralized data sources while preserving privacy.
- Meta-Learning: Comprehensive introduction to meta-learning, focusing on training models that can quickly adapt to new tasks with minimal training data.
Target Audience
This course is intended for individuals who have a solid foundation in machine learning and deep learning and are looking to specialize in advanced techniques for building and optimizing high-performance AI models. It is suitable for:
- Data Scientists
- Machine Learning Engineers
- AI Researchers
- Software Engineers with an interest in Deep Learning
- Graduate Students in related fields
Prerequisites
Before taking this course, you should have:
- A solid understanding of machine learning concepts, including supervised learning, unsupervised learning, and reinforcement learning.
- Experience with deep learning frameworks such as TensorFlow or PyTorch.
- Proficiency in Python programming.
- Basic knowledge of linear algebra, calculus, and probability.
Course Benefits
By completing this course, you will:
- Gain a deep understanding of advanced deep learning architectures and optimization techniques.
- Develop the skills to build and deploy high-performance AI models for real-world applications.
- Learn how to diagnose and address common challenges in deep learning.
- Become proficient in using industry-standard deep learning frameworks.
- Enhance your career prospects in the rapidly growing field of artificial intelligence.
- Be well-prepared to tackle cutting-edge research and development projects in deep learning.
Why this course is valuable
- The course emphasizes practical application, providing you with hands-on experience in building and optimizing deep learning models.
- You'll learn from real-world case studies and examples, gaining valuable insights into the challenges and opportunities in the field.
- The course content is constantly updated to reflect the latest advancements and research trends in deep learning.
- You'll gain a competitive edge in the job market by acquiring specialized skills in advanced deep learning and model optimization.
- This knowledge is essential for designing the next generation of AI-powered applications.
New here? Sign in to learn and earn certificates!
External Resources
How to Get Certified
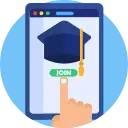
Enroll in the Course
Click the "Enroll" button to view the pricing plans.
There, you can select a plan or your preferred options and complete your payment to access the course.
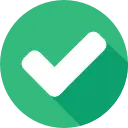
Complete the Course
Answer the certification questions by selecting a difficulty level:
Beginner: Master the material with interactive questions and more time.
Intermediate: Get certified faster with hints and balanced questions.
Advanced: Challenge yourself with more questions and less time
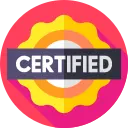
Earn Your Certificate
To download and share your certificate, you must achieve a combined score of at least 75% on all questions answered.
Course Features
Honorary Certification
Receive a recognized certification before completing the course.
Priority Support
Around-the-clock assistance for any questions or concerns you may have.
Pricing Plans
Currency
Sign in to change your currency
I'm not ready to enroll?
Our team is here to help you choose the best options for your learning goals.
Frequently Asked Questions
For detailed information about our Advanced Deep Learning & AI Model Optimization course, including what you’ll learn and course objectives, please visit the "About This Course" section on this page.
The course is offered online. If you want to meet people in person, you can choose the "Networking Events" option when you enroll. These events allow you to connect with instructors and fellow participants in person.
The course doesn't have a fixed duration. It has 17 questions, and each question takes about 5 to 30 minutes to answer. You’ll receive your certificate once you’ve answered most of the questions. Learn more here.
The course is always available, so you can start at any time that works for you!
We partner with various organizations to curate and select the best networking events, webinars, and instructor Q&A sessions throughout the year. You’ll receive more information about these opportunities when you enroll.
You will receive a Certificate of Excellence when you score 75% or higher in the course, showing that you have learned about Advanced Deep Learning & AI Model Optimization.
An Honorary Certificate allows you to receive a Certificate of Commitment right after enrolling, even if you haven’t finished the course. It’s ideal for busy professionals who need certification quickly but plan to complete the course later.
The course price varies based on the features you select when you enroll. We also have plans that bundle related features together, so you can choose what works best for you.
No, you won't. Once you obtain a certificate in a course, you retain access to it and the completed exercises even after your subscription expires. However, to take new exercises, you'll need to re-enroll if your subscription has run out.
To verify a certificate, visit the Verify Certificate page on our website and enter the 12-digit certificate ID. You can then confirm the authenticity of the certificate and review details such as the enrollment date, completed exercises, and their corresponding levels and scores.
Can't find answers to your questions?
Discussion Forum
Join the discussion!
No comments yet. Sign in to share your thoughts and connect with fellow learners.
Featured Courses
- 349 Views
- 21 Questions
- 482 Views
- 22 Questions
- 78 Views
- 16 Questions
- 269 Views
- 16 Questions
- 328 Views
- 13 Questions
- 381 Views
- 22 Questions